Using health data from smart wearables for research
For the successful treatment of many diseases, regular check-ups are essential to assess their progress and adjust the therapy. This applies, for example, to diseases of the heart such as chronic coronary heart disease (CHD), acute myocardial infarction or heart failure. These diseases are among the leading causes of death in industrialized countries. AI methods can help identify patients at increased risk of disease.
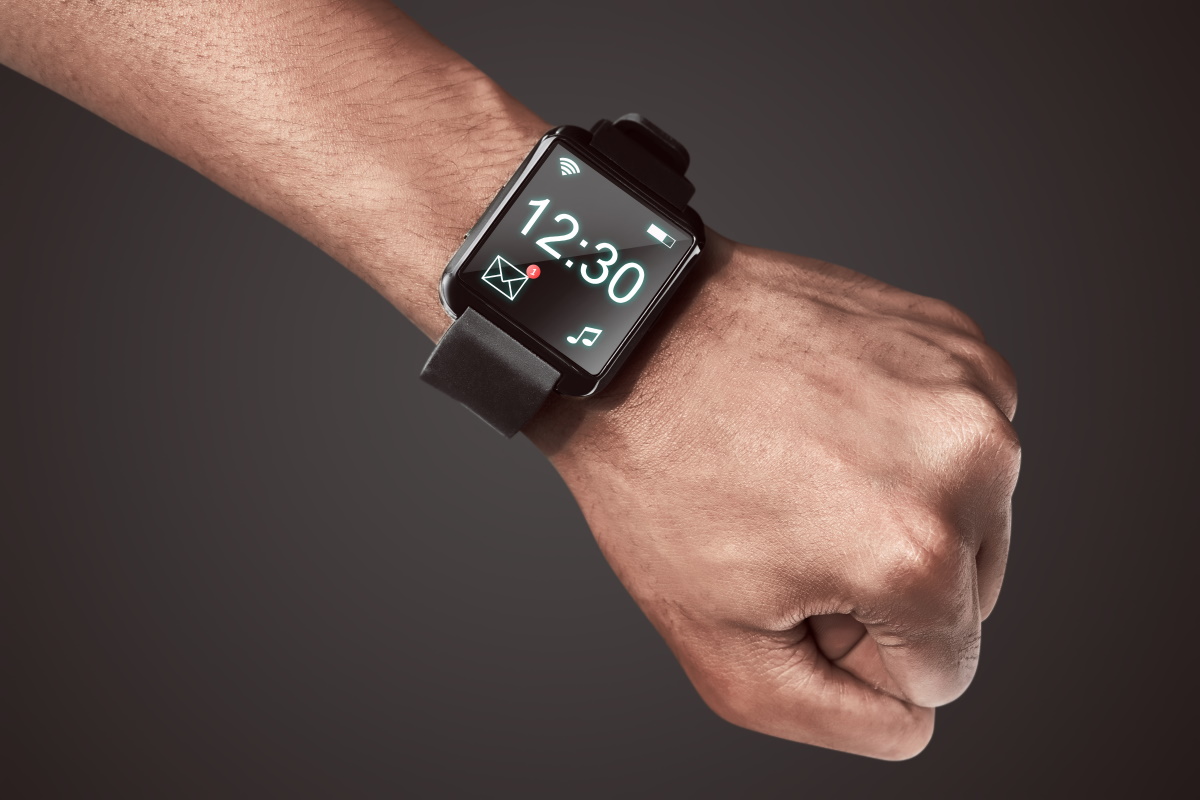
Control examinations are usually performed selectively at more or less large intervals and thus represent only a snapshot. Particularly in the case of chronic diseases that are getting progressively worse, however, it can be assumed that more frequent or even continuous monitoring would be beneficial for patients. So-called smart wearables - small, mobile devices worn on the body that can continuously record health data - promise support here.
This data promises to be a helpful supplement to other health data collected in doctors' offices or clinics, for example. In practice, however, some challenges arise: The collected data from patients are located in different data pots and currently cannot be easily merged. The reasons for this are aspects of data security and data sovereignty (especially in the case of personal data), the use of different, incompatible data formats, and requirements for the secure transfer of data between different systems.
Aggregated health data as a basis for medical AI applications.
However, merging data from different sources promises great potential for medicine: If large volumes of data are available, this enables them to be evaluated using adapted analysis methods such as research-based AI processes. These AI methods can be used to identify patients with an increased risk of disease. An individual risk profile can be determined for individual patients, on the basis of which an adapted, personalized treatment and therapy can be carried out. In order to be able to make a valid risk prediction, the AI methods must be "trained"; a sufficiently large database is essential for this.
In the BMBF-funded study "Smart Health Connect," researchers at the Berlin Institute of Health at Charité (BIH) conducted a feasibility study on integrating smart wearable data into a larger data pool. One of the core elements of the developed applications is a smartphone app through which users can transmit the health data measured by their smartwatch (e.g. ECG, heart rate) to a data portal. This data portal is connected to a hospital infrastructure, for example, and gives treating physicians access to the data. Furthermore, there is an interface for an analysis of the (anonymized) data by AI applications, which, for example, provide a risk analysis for heart diseases based on the ECG data.
Harmonization of data sources via the Gaia-X framework
The security of the data is ensured through the use of current security standards, and all regulatory and harmonization of the data is done using the Gaia-X framework. Gaia-X works to create a unified data infrastructure within Europe, an open, transparent, interoperable and trustworthy ecosystem that enables the secure and sovereign exchange of data and services. As a result, the system is open for extensions to include and process data from additional sources such as ambulatory and clinical care, but also from the research context.
The feasibility study shows that it is possible to integrate data collected via smart wearables. Cardiological data can be easily and continuously recorded via these. However, a much broader application is also conceivable. In conjunction with smartphones, population-based studies can be conducted and the data of many hundreds of thousands of participants can be collected. This can be done with greatly reduced or no personal contact with the study participants, which should lower the barriers to participation and lead to more diverse data sets than in traditional medical studies.
Application facts
- Predictive Analytics
Charité – Universitätsmedizin Berlin
University/Research Institution
Website